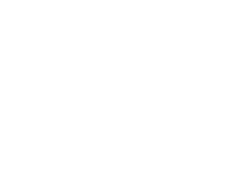
Driving Data With Emerging Technologies: Insights from GSA and DOD
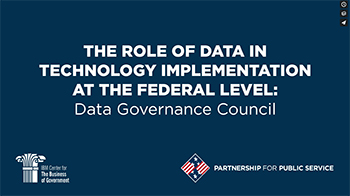
As government agencies strive to improve service by implementing advanced technologies, how that data informs strategy and business decisions represents a critical element of success. The IBM Center for The Business of Government and the Partnership for Public Service recently hosted the first of two virtual panels on this subject, focused on readying agency data by using artificial intelligence and other technology solutions. These sessions focused on detecting and addressing agency data quality issues that might stem from bias or inaccuracy, and on addressing barriers to data sharing within and across agencies to promote better access through technology. The insights and lessons learned from these discussions will help government to advance data strategies within and between agencies.
The first panel brought together Bryan Lane, Director of Data and Artificial Intelligence in the General Services Administration’s Centers of Excellence, and Alka Patel, Head of AI Ethics Policy in the Department of Defense Joint AI Center. The speakers focused on the role data plays in technology implementation and steps agencies should take to ready their data for use with emerging technologies such as artificial intelligence. The discussion focused on the importance of collecting, cleaning and organizing data.
Highlights from the full discussion, available for viewing here, follow.
The JAIC has three functions:
- Technology accelerator focused onAI.
- Technology enabler to scale Al-related technology acrossDOD.
- Department wide coordinator of AI activities.
Because the JAIC acts as a broker across a huge mission set in DOD, it has to rely on a flexible data governance structure in place to be able to support all of these mission sets.
Bryan Lane leads the JAIC Data Governance Council, which helps develop best practices for data use – including documentation, traceability, collection, or removal. The Council helps the JAIC develop tools and processes for responsible data strategies, with transparency a key element so that other stakeholders can best tap into existing data or re-use it for different purposes. Alka Patel notes that the JAIC works with the Council to incorporate AI best practices into policies across the DOD.
The Council helps address issues starting early in the data discovery phase. The Council starts with a short data discovery sprint with review of current documentation and interviews and to understand priorities. The discovery phase helps identify areas for initial action, such as data management -- identifying a data set, enabling data movement to key users, and ensuring data availability. The Council further helps the JAIC with ingesting data, enabling access for the JAIC to external data sources, and acquiring common data that can help build AI approaches. Lane notes as examples of issues raised in data discovery: “You go to a customer site to pick up data, and you don't have the hardware you need to transport it back, so now this becomes an issue that that is elevated up through the Data Governance Council.”
The Council also provides a forum for discussion about data issues, helping the JAIC to share information about standards, policies, ethics, and transparency throughout the data lifecycle.
Stakeholder Involvement in the Council
The Data Council draws input from a broad diversity of stakeholders. Lane describes, “I think one of the key aspects is having a cross functional multidisciplinary group of individuals around the table. It's really important that everyone's voice is at the table when we talk about the technology. It's no longer just a developer's responsibility, when we're talking about AI, it's everyone's responsibility.” In this manner, different voices contribute to framing uses and outcomes from data, as well as how to design protections – such an approach also points out issues early that may be of interest from an oversight perspective.
More specifically, this approach allows mission leaders to work with a broad spectrum of users to develop mission-based requirements for AI products that are then developed by engineers and security experts, working as a team with data scientists, AI ethicists, and policy analysts to bring both innovation and compliance, as well as transparency to the process. “Then,” says Lane, “as we identify issues and move data through the lifecycle, we bring other advisors in on an as needed basis.”
The stakeholder engagement also involves industry, as the JAIC engages commercial partners on an unclassified platform. This allows experimentation in networks that can be accessed outside a secure environment, with adoption inside the IC following successful testing to move software into production. Lane finds, “that makes things like supporting external projects very easy, so if something is a JAIC sponsored initiative, but the products are not developed by the Army, Air Force or whoever, we still have processes in place to provide some structure around how they make decisions about data, and then a mechanism to bring information back directly in terms of best practices.”
Incorporation of ethics into the data lifecycle
Alka Patel brings a problem- and data-centric approach to ethical AI. Depending on the sensitivity of the data and confidentiality expectation of users, her work with the Data Council is premised in building appropriate safeguards into the process – including notice/consent from users, keeping data to a minimum, explainability of algorithms, and more. Patel describes an example:
“We go through the product development aspects, and start ingesting data: with private sellers, we were … trying to capture sort of the provenance of where the data was coming from, how representative it was, and limitations of the data set, and different characteristics of it … effectively looking at the content, and then assessing the information to see how the data is still being utilized and effective or not effective or needs to be modified and so forth. If you have multiple vendors and they're labeling the data all differently, how can you try to capture that and how can you try to identify that that's the root cause of perhaps the performance issue later on?”
The Data Council’s ethics-based approach also helps with transitioning applications that were developed in the private sector for commercial use, and creating incentives for converting these applications for public sector use in an ethical and responsible way. Auditability of data is also an important consideration. This also involves continuous monitoring of AI technology and data.
Challenges in working with data
Several data management challenges emerged, including data readiness and ontology mapping. Data readiness involves understanding the quality and formats, and environment around the data, which can even include translating paper logs into a machine-readable format with context that enables sound analytics around the resulting AI-based data sets. Ontology mapping is a key implementation strategy. Lane notes, “I've been focusing many of my engineering teams on ontology … from a software development point of view, you have to abstract up and create a layer that can talk to multiple different data formats and data types. So that's become kind of a practical implementation of how we deal with different ontologies.” This allows appropriate integration of structured and unstructured data, which helps address data engineering and data integration challenges.
Another challenge relates to reviewing the data and identifying acceptable analytical parameters. This includes understanding the use case and sensitivity of the data, and subjecting the result to appropriate review. Patel notes, “If for some reason, we were getting a dataset, which was not focused on PII but might have interspersed in it, then that will get escalated and elevated” for review.
Other challenges that the Governance Council addresses include:
- Determining the right content or information to be given to the user – such as how to share, training needs, and use parameters.
- Minimizing data management burden -- such as the amount of data to ingest and cleanse vs leaving the data in home systems, since data ingest and cleansing increases resource needs due to archiving, audit and traceability requirements.
- Data bias – the type of data selected also can introduce bias.
- Risk management – controlling risks relative to benefits of data access anduse.
For more detail on these and other insights from Bryan Lane and Alka Patel, listen to the roundtable discussion here.