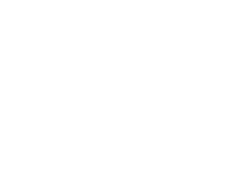
Insider Threats Part II: Current and Recommended Strategies to Mitigate Insider Threats
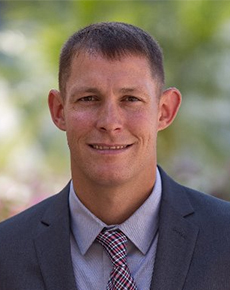
The first blog in this series presented information about Insider Threat policies and key organizations working to prevent Insider Threats. This blog will focus on current, IT-based, and recommended, whole-person risk-rating, efforts to detect and prevent Insider Threats. The next blog post will focus on the advanced analytic techniques required to continuously assess employees for potential Insider Threats.
Insider Threat Risk Reduction Plan
Before discussing some of the mitigation techniques that can be used to identify potential Insider Threats, it is important to note that any automated detection system is only a part of a larger Insider Threat risk reduction plan. That is, as part of any overarching Insider Threat program, organizations should conduct a top-to-bottom analysis of the organization to determine where and how an Insider Threat could occur. When developing their Insider Threat risk reduction plan, the organization should consider four questions adapted from a cyber intelligence perspective:
- What is an employee’s role within the organization?
- What information does that employee have access to?
- How can that employee’s access negatively affect the organization?
- What actions should be taken in the event the employee is a potential or suspected Insider Threat?
By developing their plan, organizations can identify their security priorities and the employees (or employee roles) who can most endanger their security. Importantly, the assessment enables the organization to develop strategies for preventing or mitigating an Insider Threat based on these hypothetical threats.
Five IT-related Strategies Currently Used to Mitigate Insider Threats
The effects of recent Insider Threat attacks—such as the leaks by Chelsea Manning in 2010 and Edward Snowden in 2013—illustrate the serious damage that Insiders can inflict using their access to privileged information. Because of these leaks, many current Insider Threat programs typically employ the following five IT-related capabilities:
- User Activity Monitoring: To observe and record the actions and activities of an individual accessing U.S. Government information to detect Insider Threats and to support authorized investigations. Such activity monitoring is typically conducted on electronic communications from a government employee’s computer or electronic devices.
- Data Loss Prevention: Control how users interact with data and what they can do with it. Some approaches include techniques to prohibit data from being printed, mailed, or copied to removable media, limiting the type and quantity of data that could be distributed by an Insider Threat.
- Security Information and Event Management (SIEM) and Security Operations Center: SIEM tools enable the gathering, analyzing, and presentation of information from network and security devices. An Insider Threat Program could leverage SIEM tools to correlate the network logs and provide real-time incident-based alerting to analysts within the center when a pattern is discovered. The center would then conduct an assessment and determine what actions to take, based on their Insider Threat risk reduction plan.
- Analytic Techniques: Leverage advanced data mining, machine-learning, and statistical capabilities to identify anomalous network activity, including the correlation of identities and authentication/authorization levels depending on risk levels.
- Digital Forensics Tools: Use of IT tools and techniques to investigate digital artifacts on a system or device for actions following suspected Insider Threat actions.
While these capabilities are a necessary part of any Insider Threat program, they are insufficient for implementing a comprehensive program. These capabilities are reactive in their response to potential threats. Their focus on internal information—network logs and files accessed, for example—limits the organization’s ability to proactively prevent potential Insider Threats. Moreover, these capabilities almost entirely fall within the organization’s IT administration, meaning that they are only useful in detecting IT-related Insider Threats. These techniques do not identify employees on the verge of physical harm. And because these capabilities are reactive, they do not provide management with the knowledge required to intervene before an Insider Threat occurs. In short, these capabilities do not consider all the aspects relevant to an employee’s risk as an Insider Threat.
The Whole-Person Strategy to Mitigate Insider Threats
In a 2015 report entitled Analytic Approaches to Detect Insider Threats, the Software Engineering Institute (SEI) from Carnegie Mellon University presented an analytic framework for conducting the “whole-person concept” continual evaluation of employees to detect and prevent Insider Threats. Their framework captures all the capabilities described above, but adds significantly to the list and requires the integration of internal and external information to proactively prevent Insider Threats. The SEI report decomposes the analytic requirements necessary to detect Insider Threats into three categories: Activity-Based Analytics, Content-Based Analytics, and Inferential Analytics.
Activity-Based Analytics: Activity-Based Analytics use content and event-based information derived from network sources to understand user activity. Activity-Based Analytics are decomposed into three sub-categories:
- System: Examining the changes or trends in IT asset behavior, data, or access patterns.
- Facility: Analyzing changes in the time or locality of an employee’s physical access patterns.
- Business Capabilities: Analyzing business or mission capabilities either internally or for changes and failure or externally for leaks or capability duplication.
Content-Based Analytics: The SEI report describes Content-Based Analytics as using content captured from network components and applications to examine user characteristics. Content-Based Analytics are decomposed into three sub-categories:
- Social: Analyze social interactions and communications on social networks.
- Health: Analyze network activity and content to derive potential indicators of mental health issues.
- Human Resources: Analyze network activity to indicators of external life events or complaints against the organization.
Inferential Analytics: Finally, the SEI report describes Inferential Analytics as using network content to refine the understanding of user behavior considering other information sources. In short, Inferential Analytics compare the employee’s current status to his or her historical patterns. Inferential Analytics are also decomposed into three sub-categories:
- Financial: Analyze network activity to identify indicators of unexpected changes in wealth or affluence.
- Security: Analyze network activity for indicators of security violations.
- Criminal: Analyze network sources for court or criminal activity.
By conducting analysis along these three categories, an organization can develop a regularly-updated, whole-person risk-rating score for its employees, akin to a credit score. By setting alerts to indicate high-risk employees, or employees with large changes to their scores, organizations can take proactive steps to deter or prevent Insider Threats from hurting the organization. A Defense Personnel and Security Research Centerinitiative is employing a pilot of this type of system to determine the utility of continually monitoring personnel with security clearances “through use of additional public records and appropriate social media data.” A similar methodology of combing automated records checks and public data into a scoring system has been identified as a solution for reducing the backlog of more than 700,000 applicants awaiting security clearance adjudications.
The final blog in this series will focus on key advanced analytic techniques required to implement a whole-person risk-rating system of continuous evaluation as part of an Insider Threat program, as well as the challenges to implementing such a system.
Disclaimer: The ideas and opinions presented in this paper are those of the author and do not represent an official statement by the U.S. Department of Defense, U.S. Army, or other government entity.