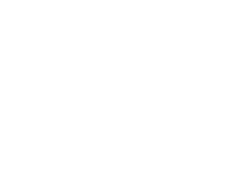
Creating Public Value using the AI-Driven Internet of Things
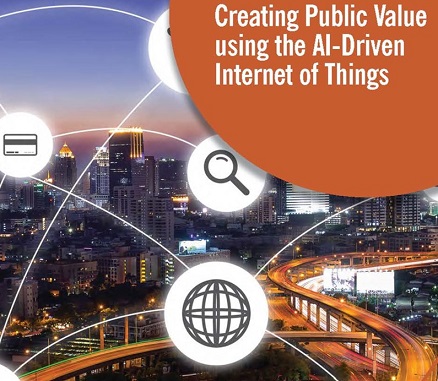
Government agencies seek to deliver quality services in increasingly dynamic and complex environments. However, outdated infrastructures—and a shortage of systems that collect and use massive real-time data—make it challenging for the agencies to fulfill their missions. Governments have a tremendous opportunity to transform public services using the “Internet of Things” (IoT) to provide situation-specific and real-time data, which can improve decision-making and optimize operational effectiveness.
In this new Center report, Creating Public Value using the AI-Driven Internet of Things, by Professor Gwanhoo Lee of American University, describes IoT as a network of physical “things” equipped with sensors and devices that enable data transmission and operational control with no or little human intervention. Organizations have recently begun to embrace artificial intelligence (AI) and machine learning (ML) technologies to drive even greater value from IoT applications. AI/ML enhances the data analytics capabilities of IoT by enabling accurate predictions and optimal decisions in new ways. Professor Lee calls this AI/ML-powered IoT the “AI-Driven Internet of Things” (AIoT for short hereafter). AIoT is a natural evolution of IoT as computing, networking, and AI/ML technologies are increasingly converging, enabling organizations to develop as “cognitive enterprises” that capitalize on the synergy across these emerging technologies.
Strategic application of IoT in government is in an early phase. Few U.S. federal agencies have explicitly incorporated IoT in their strategic plan or connected the potential of AI to their evolving IoT activities. The diversity and scale of public services combined with various needs and demands from citizens provide an opportunity to deliver value from implementing AI-driven IoT applications.
Still, IoT is already making the delivery of some public services smarter and more efficient, including public parking, water management, public facility management, safety alerts for the elderly, traffic control, and air quality monitoring. For example, the City of Chicago has deployed a citywide network of air quality sensors mounted on lampposts. These sensors track the presence of several air pollutants, helping the city develop environmental responses that improve the quality of life at a community level. As the cost of sensors decreases while computing power and machine learning capabilities grow, IoT will become more feasible and pervasive across the public sector—with some estimates of a market approaching $5 trillion in the next few years.
Although it has great potential for creating public value, its successful deployment requires a clear focus on public value, a well-formulated implementation strategy and new kinds of organizational and technological capabilities. Professor Lee’s research aims to develop a framework of alternative models for creating public value with AIoT, validating the framework with five use cases in the public domain. Specifically, this research identifies three essential building blocks to AIoT: sensing through IoT devices, controlling through the systems that support these devices, and analytics capabilities that leverage AI to understand and act on the information accessed across these applications. By combining the building blocks in different ways, the report identifies four models for creating public value:
- Model 1 utilizes only sensing capability to create new public value. Smart sensors collect data such as image, sound, light, location, movement, speed, temperature, air quality, etc., and send them to the server in the cloud.AI/ML technology can improve the accuracy of the data by using pattern recognition algorithms.
- Model 2 uses sensing capability and controlling capability to deliver new public value. Simple calculations or rules determine how to control a certain system based on the data collected by sensors. For example, simple calculations of queues of an intersection can help control the interval of traffic lights. A main benefit of the AIoT systems in this category is preventing human errors when controlling public assets.
- Model 3 leverages sensing capability and analytics capability to generate new public value. AIoT systems are not intended to control systems. Instead, the main value of this model is to support better predictions and optimal decisions. Advanced analytics methods and tools can help discover important data patterns that humans cannot easily detect due to the complexity and size of the data. AI/ML algorithms can strengthen these analytics capabilities because their accuracy improves continuously as they are trained with more data.
- Model 4 combines all three capabilities representing the most advanced and sophisticated way of producing new public value. The AIoT systems based on this model can deliver tremendous value as their operation can be continuously optimized by adapting to changing environments. The AIoT system in this category needs to sense and analyze a large volume of data and control the system in real time. AI/ML algorithms can improve the safety of autonomous vehicles by reducing critical errors as they continue to learn anomalies and exceptions.
The four models for creating value with AIoT require different levels of technological and organizational maturity.
The analysis of five AIoT use cases in the public transport sector from Germany, Singapore, the U.K., and the United States identifies 10 critical success factors, such as creating public value, using public-private partnerships, engaging with the global technology ecosystem, implementing incrementally, quantifying the outcome, and using strong cybersecurity measures. Based on these critical success factors derived from the five case studies, Professor Lee outlines a set of recommendations for government agencies considering AIoT implementation, addressing topics that include data quality, security, public-private partnerships, and reducing potential bias. These recommendations are formulated to inform leaders at all levels of government. The following is a synopsis of those recommendations
Develop a strategy for creating public value with alternative models: Considering their level of organizational readiness, governments should develop a strategy that lays out how to create public value using the four different models while managing the risks associated with them. This strategy should also include how to develop organizational and technological capabilities over time to use advanced value creation models.
Make your AIoT projects value-driven, not technology-driven: As AIoT technologies are advancing fast, it is tempting to initiate AIoT projects mainly based on new technological capabilities. Government leaders should never implement a new AIoT application without a compelling business case suggesting significant value to the public.
Experiment, validate and then scale: Government leaders should take an experiment-validate-scale approach to manage risks and uncertainties. During the process, the organization should develop not only new technological capabilities but also a new mentality and a new innovation culture because, unlike traditional IT systems, AIoT systems require physical things (as compared to software) to get installed, connected, secured, and managed. Without the right technological capabilities and innovation culture in place, experiments and pilot projects are not easily scaled to full-blown applications.
Develop a data strategy for AIoT: Governments should develop a strategy for identifying, organizing, using, and maintaining data that are relevant to their AIoT applications, while efficiently handling other data that have little value. Governments can foster innovation by making the data collected by AIoT devices accessible to the public. By doing so, governments empower citizens, companies, and researchers to create new value out of the data.
Promote Security by Design and Privacy by Design approaches: Given their responsibility to protect the public interest, governments are uniquely positioned to help develop a secure IoT. The U.S.Federal Trade Commission (FTC) published guidance on what companies should consider when designing and marketing IoT products. Governments should promote Security by Design (SbD) and Privacy by Design (PbD) and ensure that their IoT vendors are adopting these principles.
Find alternative solutions to the ‘notice and consent’ framework for privacy protection: The traditional ‘notice and consent’ framework that protects user privacy may not make sense to certain IoT devices with limited or nonexistent user interfaces. Governments should encourage, lead, and incentivize IoT manufacturers to develop alternative solutions to the ‘notice and consent’ method. For example, IoT devices can use online dashboards, apps, or customer support to increase transparency about data practices.
Leverage external resources and capabilities: As AI/ML technologies are advancing rapidly, an ecosystem around them is also quickly flourishing. Considering the limited expertise and resources that governments have, they should actively leverage that external expertise, resources, and capabilities to develop and manage AIoT applications instead of reinventing them with internal resources.
Coordinate with other government agencies: It is crucial to establish interagency coordination mechanisms for AIoT. A government agency will greatly benefit from sharing its best practices and lessons learned with other agencies. To foster interagency coordination, governments should consider creating an interagency AIoT Center of Excellence that coordinates on AIoT-related issues and promotes knowledge sharing.
Promote public-private partnership: Our analysis of the use cases suggests that a strong public-private partnership is essential for the success of AIoT. Government alone cannot do everything effectively regarding application of rapidly changing technologies. For productive public-private partnerships, the government should monitor technological development and consistently interact with the private sector.
Monitor and address errors and biases of ML algorithms: Government should develop plans for certifying ML algorithms embedded in public AIoT systems. It is therefore crucial that governments establish policies and processes to ensure that these technologies behave within appropriate and acceptable limits.
This report joins a library of IBM Center reports on topics related to AI/ML and IoT, including Innovation and Emerging Technologies in Government: Keys to Success by Dr. Alan Shark; Risk Management in the AI Era: Navigating the Opportunities and Challenges of AI Tools in the Public Sector by Justin Bullock and Matthew Young; More Than Meets AI: Part II, Improving Outcomes in Government through Data and Intelligent Automation, The Rise of the Sustainable Enterprise: Using Digital Tech to Respond to the Environmental Imperative, and Managing Cybersecurity Risk in Government by Anupam Kumar, James Haddow, Rajni Goel; and Delivering Artificial Intelligence in Government: Challenges and Opportunities by Kevin Desouza.
Professor Lee’s report provides a new and important roadmap for governments at all levels to take advantage of the promise that AI and IoT hold for improving public services and operational effectiveness.