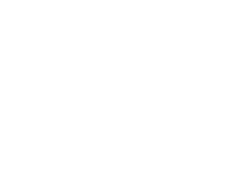
Big Data: It’s About Complexity, Not Size
Big data should not be defined as “big” based on the size of the data alone. As defined by an important Commission on Big Data, big data is “a phenomenon that is a result of the rapid acceleration and exponential growth in the expanding volume of high velocity, complex and diverse types of data.” Organizations that do not necessarily have a large volume of data can benefit from a better understanding of the art of the possible with the new generation of analytic tools designed for big data.
Lieutenant Colonel Josh Helms is a data analyst in the Army serving as a Research Fellow in the Army's Training With Industry program, through which he works with IBM for one year before returning to the Army. His research fellowship is intended to help him learn how industry analyzes big data and communicates strategic insights to senior leaders to take this knowledge base back to the Army. LTC Helms is working with the IBM Center for several months; this is the first in a series of blog posts in which he discussed research designed to address the challenges that the government faces in regards to big data.
Background
I started my big data research by taking courses at Big Data University (www.bigdatauniversity.com), an online forum provided by a community of open source enthusiasts, academia, and industry. Most courses are offered free of charge and are developed by experienced professionals and teachers. The courses include hands-on labs that students can perform on the Cloud, on VMWare images, or by locally installing the required software. Additionally, I interviewed several of the leaders within IBM’s big data and analytics community to get insights on the development of big data tools and lessons learned from business cases. I also interviewed several leaders in government to learn what big data challenges the public sector faces and how some organizations have addressed these challenges. Lastly, I invested time to read other blogs and reports on big data and analytics in the public and commercial sectors.
Where to Begin?
When starting this research, I lacked a good understanding of the meaning of big data. As I talked to colleagues about my career opportunity in this fellowship, I learned that there are many data analysts who do not have a common view of what big data is either. Many analysts who claimed to understand big data stated that they do not use or have access to big data, yet they felt industry trends pushing in that direction. I have since learned that big data is more about the complexity of the data, rather than the size of the data alone. Based on those initial discussions, the focus for this first blog entry is to help provide a better understanding of big data.
Five Dimensions of Big Data
One common misconception is that big data represents a technology. All research colleagues were clear that big data is an event or an occurrence. Andras Szakal, Vice President and Chief Technology Officer for IBM US Federal, referenced a report from TechAmerica Foundation’s Big Data Commission, “Demystifying Big Data,” which defines big data as “a phenomenon that is a result of the rapid acceleration and exponential growth in the expanding volume of high velocity, complex and diverse types of data.”
Formally, the Commission classified big data based on four dimensions, known as the Four Vs:
1. Volume – the quantity, scale, or size of the data
- ~ 2.3 trillion gigabytes of data created every day
- Most US companies have at least 100 terabytes of data stored
2. Velocity – the speed of the data and analysis; real-time analysis of streaming data
- Modern car has ~100 sensors
- NYSE captures ~1 terabyte of trade information during each trading session
3. Variety – the different forms of data; structured, semi-structured, and unstructured
- ~30 billion pieces of content are shared on Facebook every month
- Over 4 billion hours of video watched on YouTube each month
4. Veracity – the uncertainty as to the truthfulness of the data
- 1 in 3 business leaders don’t trust the information they use to make decisions
- Poor data quality costs the US economy ~$3.1 trillion a year
Brian Murrow, a leader in IBM’s Business Analytics and Strategy team, added a fifth “V”: Value, gained through analysis of the big data. He says the focus should not be on the size or volume aspect of data. Big is relative, as the complexity of the data might push the analyst to the new generation of analytic tools developed to mine the valuable information from big data. As a concept, value is a theme that reverberated through my research.
Getting to the Data You Want to Analyze
In March of 2012, Steve Mills, a Senior Vice President and Group Executive at IBM, said that “data should be thought of as a new natural resource.” He cautioned that just having massive amounts of data is not sufficient -- in fact, without the proper analytic tools, it can lead to information overload.
Tim Paydos, the Director of IBM’s World Wide Public Sector Big Data Industry Team, believes that big data is a term du jour, similar to “dot.com” in the late ‘90s. Successful businesses still practice those e-commerce principles that helped companies rise to prominence, so the dot.com has become mainstream. Those I interviewed speculated that the same will be true with the term big data over the next decade. Mr. Szakal said that “big data is about getting to the data you want to analyze. If you don’t have big data, that doesn’t mean you cannot benefit from the advanced analytic tools and processes.” He believes that organizations can get more value out of data by getting away from solely visualization and descriptive analytics, migrating towards the prescriptive and cognitive end of the analytic spectrum and automating business processes.
New Analytic Tools
Instead of focusing on the size of the data, Mr. Paydos recommends that leaders start by considering business or mission requirements to determine if they have a need for big data analytic tools. With the development of the next generation of analytics over the past decade, leaders can make a compelling case to move to big data analytics. After understanding the art of the possible, and assessing their current capabilities against identified mission requirements, they can move iteratively to fill those analytic capability gaps. Mr. Paydos believes that leaders should seize the opportunity, “acting tactically within a strategic framework with respect to needs and capability shortfalls.”
These new analytic tools enable organizations to:
- analyze all data instead of small subsets of data
- analyze data as is, cleansing as needed instead of cleansing before analysis
- explore all data and identify correlations, instead of starting with a hypothesis and selecting data to confirm or deny a hypothesis; and
- analyze data in motion/real-time, instead of waiting for it to be processed and placed in a data warehouse.
Harnessing Big Data to Make a Difference
While many analysts and leaders may believe they do not use or have access to big data, I urge them to expand their perspective on big data beyond just the dimension of volume. Agencies will benefit by assessing the holistic complexity of the data, even considering data sources that might have been deemed unusable before, and gaining a better understanding of the art of the possible with the new generation of analytic tools. So that the path not become overwhelming and cost prohibitive, organizations should develop a strategic road map to migrate towards new tools. Mr. Paydos wisely surmises: “while big data will be transformational and revolutionary, the path to harnessing big data is evolutionary and iterative.”
In future Big Data Blog Series, I will cover:
- Best practices for data governance and building trust in the data (Part One and Part Two)
- Areas in which big data has made a difference in the public sector
- Best practices for changing an organization’s culture to embrace big data
***The ideas and opinions presented in this paper are those of the author and do not represent an official statement by IBM, the U.S. Department of Defense, U.S. Army, or other government entity.***